In the final installment of our series with OMF directors, we bring you an illuminating interview with Wenzhong Xiao, PhD, Director of OMF’s Computational Research Center for Complex Diseases. Dr. Xiao performs data analysis for many of OMF’s clinical studies in addition to knowledge-based network analysis.

The Heart of the Matter
- OMF’s Computational Research Center for Complex Diseases, directed by Wenzhong Xiao, PhD, performs data analysis for many of OMF’s clinical studies in addition to knowledge-based network analysis.
- The team develops tools useful for analyzing different data types, including AI natural language processing for electronic health records and algorithms for proteomics.
- Their knowledge-based network analysis approach pulls together results from separate studies and those conducted on similar diseases to help guide future research directions.
- The majority of the computation team’s work lies in the “Data Analysis” stage of the research process.
OMF’s Computational Research Center for Complex Diseases, directed by Wenzhong Xiao, PhD, is uniquely positioned to combine data from within and outside of OMF’s collaborative centers to try to gain a holistic understanding of mechanisms of ME/CFS and related conditions, identify diagnostic and predictive biomarkers, and discover potential treatment targets. To this end, the team performs analysis for many of OMF’s hypothesis-driven clinical studies in addition to their computation-driven work.
During analysis, Dr. Xiao’s team manages a wide variety of data types, including electronic health records (EHR), surveys, genomic sequencing, proteomics, metabolomics, and more. The computational tools used to analyze these data are often specific to the type of study or data being evaluated…..
For example, the team develops algorithms that analyze the thousands of data points they get from proteomics. Whereas, when looking at EHR data, they use artificial intelligence and natural language processing to parse through the information.
In addition to analyzing data in the context of a clinical study, the computation team drives new studies that are otherwise not possible to conduct. The AI tool they developed for analyzing EHR data, for example, facilitates in silico (computer-based) trials, in which they can use patient records to retrospectively evaluate potential benefits seen from different treatments.
These tools also help the team try to overcome some of the traditional challenges seen with research on ME/CFS and related conditions. Given the funding limitations that we all know far too well, studies on ME/CFS often have smaller sample sizes and are forced to limit analysis to fewer data types than would be ideal. Combined with an already heterogeneous patient population, it can be difficult to draw useful or reliable conclusions from these studies when the data is considered on their own.
OMF’s computation team uses a knowledge-based network analysis approach to address these issues, trying to make sense of the available data. Network analysis integrates separate studies and incorporates information from other diseases to provide insight into ME/CFS and related conditions. Ultimately, this work will help prioritize studies and the data collected given limited resources.
Dr. Xiao’s team is intimately involved in the “Data Analysis” stage for many of OMF’s projects, in addition to their network medicine work analyzing data from disparate sources.
Click Here to Read the Video Trancript
Video Transcript
Dr. Meadows: Hi everyone, and welcome back to the final video in our series of interviews with OMF’s directors. Today, I am thrilled to be joined by the Director of our Computational Research Center, Dr. Wenzhong Xiao. Dr. Xiao and his team are in an exciting point at the Computation Center as they’re in a unique position to kind of be able to pull together information from our other research centers, but also outside sources to really try to glean a more holistic picture of what’s going on in ME/CFS. So welcome, Dr. Xiao.
Dr. Xiao: Thank you, Danielle. It’s great to have this opportunity to speak to the patient community.
Dr. Meadows: So today’s interview is going to be a little bit different than some of the previous videos that I’ve done with other directors and that I want to talk to you, Dr. Xiao about sort of two different styles of work that you do. So one being analysis for hypothesis-driven work, and then the other being more computation-driven work. So first if we can chat a little bit about some of the tools that you use to develop and help pull together all of the siloed data in ME/CFS, we can maybe start there. So can you give a brief overview of some of the tools that you use, maybe like artificial intelligence when analyzing data for a study?
Dr. Xiao: Sure! So I’ve been having the opportunity to have the team working on ME/CFS and related diseases for the past few years, starting I think around 2016 in collaboration with Open Medicine Foundation. The studies that we do in collaboration with other research labs try to understand essentially the mechanism of ME/CFS and related conditions, and try to identify so called diagnostic and predictive biomarkers. The predictive biomarkers are those ones that potentially can be used in evaluating the efficacy of treatments as well as potential candidates for targeting in treatments.
So the studies that we are involved range from patient electronic health records, patient surveys of symptoms and treatments, to the other end, a lot of molecular studies such as genetic sequencing, proteomics, metabolomics, epigenetics, cellular and some of the other molecular analysis.
The tools that we use are sort of specific to each one of these studies. For genetic studies, for example, that involves a lot of sequence analysis. So that’s sort of the backyard of bioinformaticians where you learn from college or graduate school the fast and efficient ways of comparing these days billions of sequences in an affordable way.
So that’s what we do when we try to, for one, try to identify genetic variants in, for example, severely ill patients that we had whole genome sequencing and try to see whether those variants might contribute to the disease process.
I think we just had a preprint out, actually today, and another project that we’re working on is to try to see whether we can identify some of these autoantibodies in ME/CFS and related diseases, and see whether that come from so called molecular mimicry. Namely, they’re microbes that would share similar sequences as sequences in the human proteome. So that involves many, many alignments of the existing microbe sequences with human proteome. So that’s the type of analysis that we typically do.
And when we study obviously proteomics, you get into this situation where proteins can be modified. So you would either develop algorithms or adapt algorithms to similarly try to conduct computation in an effective way.
And after we identify the significant, which we call disease signature, then the question is how we can make sense of it. Classically, people would use gene ontology or pathway analysis to essentially group these significant genes or proteins or metabolites into pathways and see which pathway is enriched. It’s a problem in bioinformatics that people recognize since the 80s and 90s.
And we had the opportunity to work with a group of students at Stanford in the late 1990s and early 2000 and set up a system that’s currently used by a lot of people in research world to sort of look at beyond genes and pathways, but also the genetic regulations, the metabolic regulations, and try to leverage data from other studies into this analysis. We call this knowledge-based network analysis.
So that’s the typical work that we do for molecular studies. At the other side, when we look at the electronic health record of patients these days, as you mentioned, because of AI and machine learning, a lot of large language model-based tools and so called natural language processing tools are used to extract information from the electronic health records of the patients. So we can correlate that with for example, treatments and symptoms of patients and try to see, we call these days in silico clinical trials where you basically look at patients that have equal chance of taking a particular drug but indeed took it versus those patients that have equal chance of taking that particular drug but did not take it, and then analyze whether there’s a significant difference in terms of patient symptoms and then suggest use that information as so called real-world evidence to prioritize treatments for ME/CFS and related disease.
Dr. Meadows: That’s great, thank you for that explanation. So maybe is it safe to say that when we think about different types of data that are collected. So you mentioned a lot of the omics data, for example, you use algorithms that you develop in order to actually analyze those data. And then on the electronic health records side of things, often you’ll use things like AI to help kind of make sense of all the clinical notes and all the things that are incorporated in the EHR, and then kind of combining those different, you know, data types in the analysis that you’ve done, you then extend that through network analysis, bringing in, you know, data from other sources to be able to really try to identify the most promising treatment targets. Does that sound right?
Dr. Xiao: Yes, absolutely. Excellent summary.
Dr. Meadows: Great. And so then I want to maybe expand a little bit on the network medicine side of things, network analysis side of things. So can you talk a little bit more about that process and you know how what that looks like to somebody from the outside?
Dr. Xiao: Sure, sure, absolutely. So through this, you know, analysis of multiple data sets, one of the things that we realized is that probably a lot of people in the community know this before we realize this, that compared to many other diseases, ME/CFS is understudied. Meaning that when we compare the so called sample sites of a typical study of ME/CFS with some of these well-studied diseases being cancer or heart disease, the sample size is much smaller.
And not only that, we don’t typically have the opportunity to have so called multiple-level or multiomics study on the same patients. I think largely because of the limitation of funding. So a lot of studies only look at one dimension, either genetics or just proteomics or metabolomics of the patients.
So, some of those traditional tools to leverage this multiple level of information on the same patients, and their clinical information, unfortunately, can’t be directly applied to the studies in ME/CFS yet. And on top of that, there’s significant differences often in the data sets collected by individual studies, presumably because of the heterogeneity of the disease. That’s part of the complexity of this disease.
So, instead we thought that perhaps an alternative approach at this moment is to integrate, instead the studies that we have seen so far, with studies that have been collected for other diseases as well as the findings. Or again, this knowledge-based idea of other diseases.
And the thought process is that if we can identify diseases that are well-studied but look similar to ME/CFS, perhaps we can then look at those set of diseases and see what’s common there. And perhaps that suggests to us what would be the disease system, either it’s neurological problem or metabolic problem, or particular pathways or genes that can be prioritized for our studies, given the limited resources that we have for ME/CFS.
So, that’s what we’ve been focusing on for the past couple of years. And we try to push this process one step further. That is, if we indeed identify the set of diseases that are similar at multiple levels to ME/CFS. Perhaps we can even consider some of the drug treatments that are shown to be effective in these similar diseases. So that’s something that’s ongoing at this moment, and obviously it would require verification and the clinical trials to test whether those predictions are indeed true.
Dr. Meadows: That’s great. So, in a way, you’re able to try to overcome some of the challenges that we see with ME/CFS in general, and that there’s heterogeneity in the population. And so many, you know, studies aren’t able to look at all of the things we would love to look at, you know, in one small study.
And so you’re able to kind of use some of the things that we see in similar diseases, comorbid conditions, things like that, and try to pull out some similarities to identify the best drug target.
Dr. Xiao: Exactly.
Dr. Meadows: Okay, thank you. And you’re able to also look from across varying levels as well. So you can look at, you know, the genes, but also proteins, transcriptomics, you can look at metabolomics, all those different kinds of things and pull them all together.
Dr.Xiao: Yeah
Dr. Meadows: That’s wonderful! All right, so I think with that, we’re going to wrap up the short interview today. So, I just want to thank you so much for your time today, Dr. Xiao.
Dr. Xiao: Absolutely. It’s very nice speaking with you.
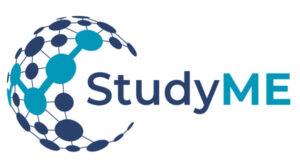
OMF StudyME is a free global recruitment tool that connects individuals interested in participating in research studies with the researchers conducting them. Join OMF StudyME, and let’s show the world how many people want a cure.
Your support helps make this groundbreaking research possible. Consider making a donation today to advance our research efforts.